Credit scoring faces several challenges in today’s rapidly evolving financial landscape. The primary issues include Bias and Fairness, Explainability and Transparency, Data Quality and Availability, Data Privacy and Security, and Technological Integration. To address these challenges, we developed the Artificial Intelligence-based Triangulated Credit Application Scorecard (ATLAS) Model. This innovative model tackles these challenges and risks, at the same time surface opportunities for greater revenue and better portfolio.
Bias and Fairness
Algorithmic Bias: AI and machine learning models can inadvertently perpetuate biases present in the training data, leading to unfair credit decisions.
Discrimination: There is a risk of discrimination based on race, gender, or socioeconomic status if the models are not carefully monitored and adjusted.
ATLAS Solution: The algorithmic biases that may arise are self-correcting or self-healing. In a typical scorecard, redeveloping and recalibrating a scorecard can take up from six months to a year. It would involve a long process and bulk data for validation. With ATLAS, calibration can be achieved in a minimum of a month or the loan’s shortest credit cycle. This allows for quick adjustments to incorrect discrimination. Additionally, there is no need for manual adjustments as the system is self-healing. For example, if a person living in a certain area received a low score or minimal likelihood of approval due to historical data of delinquency and difficulty in collection, the new good accounts in nearby areas or as a second address can soften the said historical bad records. Another example of self-correcting mode is the score weight adjustment. Applications that were given low-risk scores but become bad accounts or high-risk scores that turn out to be good payers, will trigger prominent parameters weights to adjust accordingly. The solution can be product-specific, allowing for fine tuning to vehicle loan or personal loans or any other products.
Explainability and Transparency
Complex Models: AI and machine learning models can be complex and difficult to interpret, making it challenging for lenders to explain their decisions to borrowers.
Lack of Transparency: The opacity of some credit scoring models can lead to a lack of trust among consumers and sales personnel.
ATLAS Solution: The model is triangulated and based on the 5 C’s of Credit, Social Score, and Psychometric Score. The 5 C’s of credit are Character, Capacity, Capital, Collateral, and Conditions. The social score is based on the person’s social connections, this is determine via social media account and membership in organizations. The psychometric score is derived from the applicant’s responses to crafted questions. It will determining their priorities and attitude towards obligations. The psychometric and social scores can be easily understood as they are based on personal values and social connections which sales and applicants can relate. Further, to improve understanding, scoring models will be explained to sales personnel and consumers in layman’s terms. Videos in commonly used languages and examples in the provided materials will be made available. The service is available 24/7 with technical support, including a chat bot. Suggested scripts for rejected applications will be provided and can be modified or used as is by the credit or sales team.
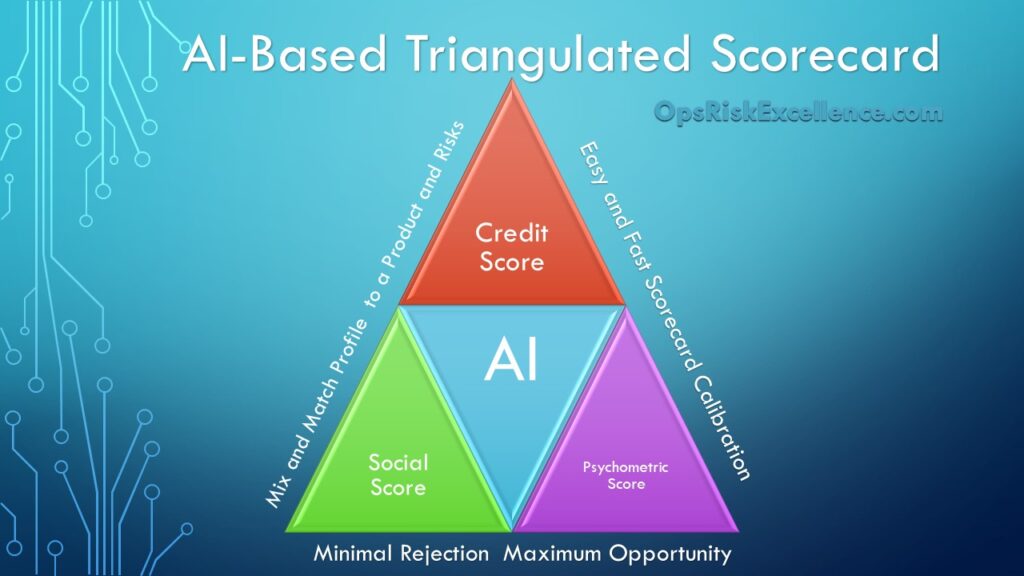
Data Privacy and Security
Data Breaches: With the increasing amount of data used in credit scoring, the risk of data breaches and cyberattacks has grown. Protecting sensitive information is a major concern.
Regulatory Compliance: Ensuring compliance with data protection regulations, such as the General Data Protection Regulation (GDPR), is essential but challenging for credit scoring agencies.
ATLAS Solution: The scorecard is primarily designed to comply with GDPR and country-specific data privacy laws. A significant checklist must be completed before scorecard installation. Necessary controls include Data Encryption, Access Control, Regular Audits and Monitoring, Data Minimization, and a Response Plan. In addition to these external controls, the scorecard has an embedded algorithm to detect the likelihood of fraud. The organization’s historical fraud events can be mined to determine the likelihood of fraud based on the documents and data submitted.
Data Quality and Availability
Incomplete Data: Traditional credit scoring models often rely on limited data, resulting in inaccurate assessments for individuals with thin credit files.
Alternative Data: While using alternative data sources can improve accuracy, it also raises questions about the reliability and relevance of such data.
ATLAS Solution: The scorecard complies with GDPR and country-specific data privacy laws. A significant checklist and requirements must be completed before scorecard installation. The necessary controls includes, but not limited to the folllowing: Data Encryption, Access Control, Regular Audits and Monitoring, Data Minimization, and a Response Plan. Additionally, the scorecard has an embedded algorithm to detect the likelihood of fraud.
Technological Integration
Integration Challenges: Implementing AI and machine learning models into existing systems can be complex and resource-intensive.
Operational Efficiency: Ensuring that new technologies improve operational efficiency without causing disruptions is a significant challenge.
ATLAS Solution: The scorecard results can be easily fetched and integrated into the existing system via an embedded API.
The ATLAS solution does not only address key challenges but also factors the residual value of collateral and its fluctuations based on scraped data from the internet. Origination profitability is also computed alongside the score.
For a demonstration, please contact admin@opsriskexcellence.com or JDioneda@gmail.com.